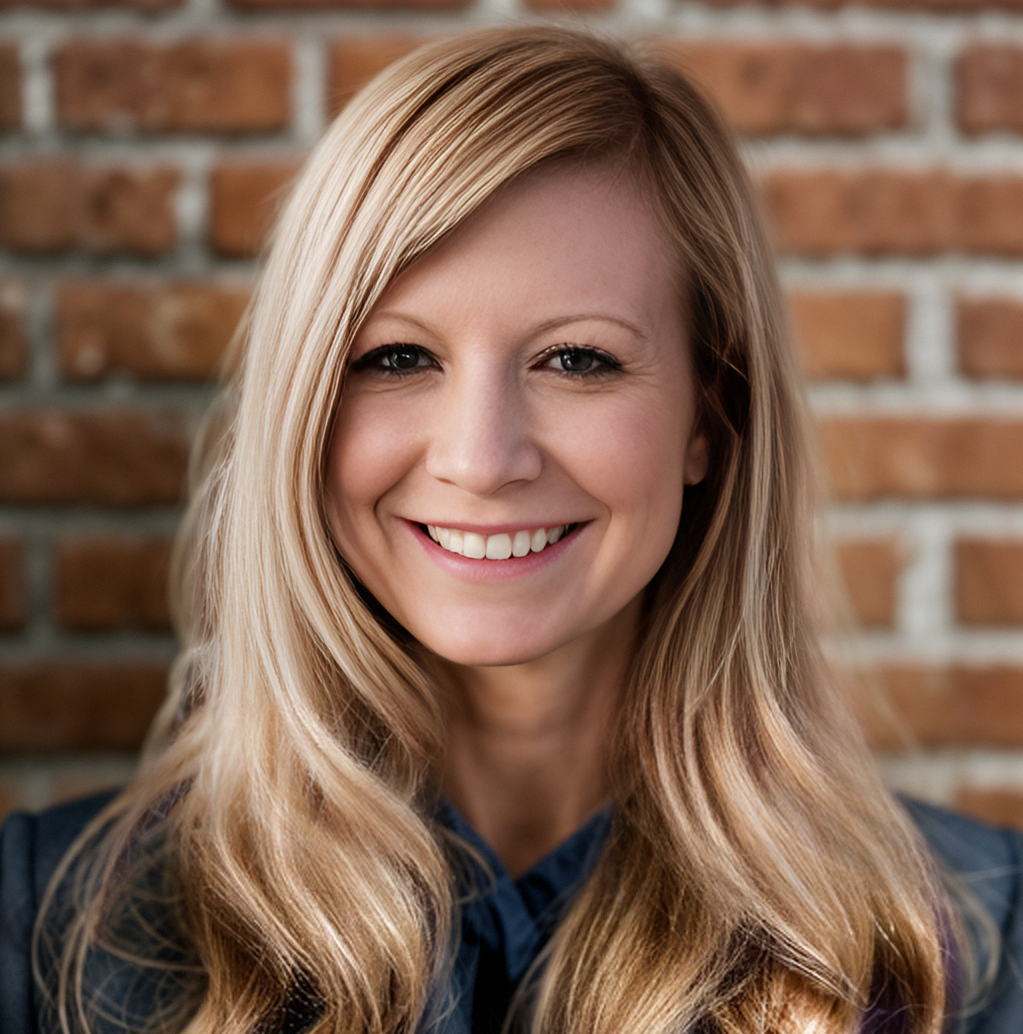
Krystal Welland
Krystal Welland is Silberman Lawâs Lead Statistician. Krystal performs complex statistical analyses related to pay equity, affirmative action, adverse impact, and DE&I. Krystal works closely with the firmâs clients to develop meaningful, practical and strategic analyses that help employers address pay gaps, enhance EEO and OFCCP compliance, and move the needle on diversity. Krystal regularly presents at local, regional, and national ILG conferences.
Monday, July 29
Workshop 2
Mitigating âCampus-Like Settingâ Audit Risks: Strategic Lessons from the Trenches
Chris Chrisbens and Krystal Welland, Silberman Law PC
OFCCPâs review of multiple âcampus-like settingâ AAPs fundamentally changes OFCCP audits and increases audit risk. The presentation learning objectives include:
- How OFCCPâs shift to campus audits impacts audit risk
- What to expect from OFCCP
- Specific steps to mitigate risk by adapting our audit preparation â particularly data aggregation
We will discuss strategic AAP and job group structures, analysis of aggregated data, as well as how supporting documents may help mitigate risk.
Wednesday, July 31
Workshop 1
An I/O Psychologist, Labor Economist and Statistician Walk into a (Proverbial) Bar and Discuss Pay EquityâŚ
Quenton Wright, Charles River Associates; Joanna Colosimo, DCI Consulting Group Inc.; Krystal Welland, Silberman Law PC
Join a panel discussion between an I/O Psychologist, Labor Economist and Statistician addressing the top five most challenging analytical topics in todayâs landscape. The panelists will share firsthand experiences and hard-earned advice. Attendees will obtain best practice recommendations on:
⢠Strategic analysis aggregating compensation snapshots
⢠Providing OFCCP proof of a âpay evaluationâ
⢠Analyzing and submitting state specific pay data
⢠Determining pay analysis groups
⢠Assessing whether an explanatory factor is biased